DOI: 10.1016/j.ridd.2011.09.004
Authors:
Róbert Bódizsa,b, Ferenc Gombosb, and Ilona Kovácsb,c
aInstitute of Behavioural Sciences, Semmelweis University, Nagyvárad tér 4, Budapest, Hungary
b HAS-BME Cognitive Science Research Group of the Hungarian Academy of Sciences, Egry József u. 1, H-1111, Budapest, Hungary
c Department of Cognitive Sciences, Budapest University of Technology and Economics, Egry József u. 1, H-1111, Budapest, Hungary
Abstract
Sleep EEG alterations are emerging features of several developmental disabilities, but detailed quantitative EEG data on the sleep phenotype of patients with Williams syndrome (WS, 7q11.23 microdeletion) is still lacking. Based on laboratory (Study I) and home sleep records (Study II) here we report WS-related features of the patterns of antero-posterior 8– 16 Hz non-rapid-eye-movement (NREM) sleep EEG power distributions. Participants in Study I were 9WS and 9 typically developing (TD) controls matched for age (14–29 years) and sex, and sleeping for two consecutive nights in the laboratory. WS participants were characterized by region-independent decreases in 10.50–12.50 Hz and central increases in 14.75–15.75 Hz EEG power. Region-independent decreases and increases in z-scores of the spectra were observed in the 10.25–12.25 Hz and 14–16 Hz ranges, respectively. Moreover, in the EEG spectra of participants with WS a lower probability for the emergence of a frontally dominant peak was observed. Parietal fast sigma peaks and the antero-posterior shifts in power distributions were of higher frequencies in WS (1Hz difference). A 1 year follow-up of 9 WS and 3 TD participants, as well as their inclusion into larger samples (20 WS and 20 TD, age: 6–29 years) of a two-night ambulatory home polysomnography study confirmed the WS-specific decrease in alpha/low sigma power (8–11.75 Hz) and the pattern of z-score differences (decreases: 8.50–11.25 Hz; increases: 13.5–14 Hz), including the antero-posterior shifts in power distribution (0.5 Hz) and some features of the spectral peaks. Altogether these data suggest a decrease in alpha/low sigma power, as well as a redistribution of NREM sleep 8–16 Hz EEG power toward the higher frequencies and/or a higher frequency of NREM sleep thalamocortical oscillations in WS. 2011 Elsevier Ltd. All rights reserved.
Keywords:
Williams syndrome, Sleep EEG, Sleep spindles, Cortical synchronization, NREM
1. Introduction
Evidence supports the robustness and stability of individual differences in non-rapid eye movement (NREM) sleep EEG spectra with a special emphasis on the 8–16 Hz range corresponding to alpha and sigma activity (Buckelmu¨ ller, Landolt, Stassen, & Achermann, 2006; De Gennaro, Ferrara, Vecchio, Curcio, & Bertini, 2005; De Gennaro et al., 2008; Tan, Campbell, Palagini, & Feinberg, 2000; Tarokh, Carskadon, & Achermann, 2011). Data supporting this stability were provided by period and amplitude analysis measures of several EEG bands including sigma activity, which were shown to be highly consistent across nights (Feinberg, Fein, & Floyd, 1980). The particularly high inter-night correlation in the sigma band power reported by Tan et al. (2000) was attributed to the marked and stable individual differences in phasic spindle activity (Gaillard & Blois, 1981; Silverstein & Levy, 1976). A substantial genetic influence on spectral composition of NREM sleep in humans, in particular with respect to alpha and sigma frequencies was also reported (Ambrosius et al., 2008). Furthermore, the unique profile of the 8–16 Hz EEG spectra of NREM sleep was considered as the EEG fingerprint of sleep (De Gennaro et al., 2005), and found to be the one of the most heritable human traits, characterized by a heritability estimate of 96%, not influenced by sleep need and intensity (De Gennaro et al., 2008). A similar invariance of EEG power distribution during human NREM sleep has also been suggested by a full-scalp recording (Finelli, Achermann, & Borbély, 2001). The NREM sleep EEG spectral profiles and their topographical distributions were hypothesized to reflect genetically determined traits of functional neuroanatomy rather than sleep-dependent mechanisms (De Gennaro et al., 2005; Finelli et al., 2001). Given the increasing evidence for the robustness of this trait-like feature of NREM sleep EEG, further studies unravelling the distinct phenotypes peculiar to specific developmental disabilities are of potential interest. Indirect evidence for the promising nature of this approach comes from the studies reporting alterations in sleep EEG spindling of patients with Asperger syndrome (Godbout, Bergeron, Limoges, Stip, & Mottron, 2000), developmental dyslexia (Bruni et al., 2009) or malformations of cortical development (Selvitelli, Krishnamurthy, Herzog, Schomer, & Chang, 2009). Although, several phasic spindle features were shown to be inherently related to the individual shape of the power spectra in the alpha/sigma band (Bódizs, Körmendi, Rigó, & Lázár, 2009), no study focusing on developmental disorders characterized the fine structure of the genetically determined 8–16 Hz NREM sleep EEG. However, some distinct features of sleep spindling were characterized in patients with malformations of cortical development (Selvitelli et al., 2009).
Williams syndrome (WS) is a genetically determined developmental disorder linked to a microdeletion of 25–28 genes in chromosome 7q11.23 and characterized by mild to moderate mental retardation, learning difficulties, cardiovascular abnormalities, high sociability and empathy and a distinctive cognitive-linguistic profile (Järvinen-Pasley et al., 2008; Meyer- Lindenberg, Mervis, & Berman, 2006). Perhaps this latter aspect is the most interesting for cognitive research: severe visualspatial deficits and relative strength in expressive language (Bellugi, Lichtenberger, Jones, Lai, & St George, 2000; Karmiloff- Smith et al., 1997). As overactivity and short attention span is typical inWSmore than 50%ofWS individuals are diagnosed with attention deficit hyperactivity disorder (Leyfer, Woodruff-Borden, Klein-Tasman, Fricke, & Mervis, 2006; Morris & Mervis, 2000). Available data on the sleep of childrenwithWS suggest decreases in sleep time, sleep efficiency, and REMtime aswell as increased slow-wave sleep (SWS) and periodic leg movements during sleep (PLMS) (Arens et al., 1998;Mason, Arens, Sharman, Pack, & Kaplan, 2008). Actigraphic and polysomnographic studies support the persistence of some of these early age sleepfeatures ingroups ofadolescents andyoungadultswithWS, including increasedSWS, decreasedREMsleepandsleepefficiency, but not the diagnosis of PLMS (Goldman, Malow, Newman, Roof, & Dykens, 2009; Gombos, Bódizs, & Kovács, 2011). Given the genetic determination of both WS and the 8–16 Hz NREM sleep EEG, as well as the relevance of studying polygraphic sleep in neurodevelopmental disabilities, our aim is to unravel the peculiarities of the individual sleep EEG fingerprints ofWSparticipants. Results of these analyses might shed light on both the neural correlates ofWSand the genetic factors determining sleep EEG in general. We hypothesize that there are specific features of the individual sleep EEG fingerprint in WS and that the distribution of NREM sleep 8–16 Hz EEG power along the antero-posterior cortical axis distinguishes WS from typically developing (TD) participants.
2. Study I: sleep EEG fingerprints according to laboratory sleep recordings of participants with WS
2.1. Methods
2.1.1. Participants
Nine WS and 9 age- and gender matched TD control participants were enrolled in the study. WS participants were recruited from the Hungarian Williams Syndrome Association. WS diagnosis was based on the fluorescence in situ hybridization test of the absence of an elastin gene from chromosome 7. Exclusion criteria for TD participants were medical diagnosis of sleep problems or psychiatric, neurological or other medical disorder. Body mass index (BMI) was in the normal and under the normal range in theWSgroup (between 13.9 and 26 with an average of 19.4) and in the normal range in the TD group (between 17.4 and 23.3 with an average of 20.5). The research protocol was approved by the Ethical Committee of the Budapest University of Technology and Economics. Adult participants or the parents of the underage participants signed informed consent for the participation in the study according to the Declaration of Helsinki. Participants were free of drugs except for 1WS patient who was on stable medication with clonidine (150 mg/day), enalapril (5 mg/day), acetylsalicylic acid (500 mg/day), betaxololi hydrochloridum (20 mg/day), and amlodipine (15 mg/day). Those patients not on medication were not withdrawn from any pharmacological treatment prior to the study. Age- and sex-matching of TD participants were performed in a case-controlled manner: every WS subject had a TD pair with similar age (within the limits of 2 years, but usually less than 1 year difference) and sex. One except in sex-matching was intentionally introduced in the case of a 16 years old dizygotic twin pair discordant for WS and sex, the non-WS 16 year old girl serving as a TD control of her WS brother. Age range of the whole sample was 14–29 years, the mean age being 20.44 years. Five males and 13 females participated in the study.
2.1.2. Procedures and recordings
Participants underwent polysomnographic examinations on two consecutive nights during their sleep in the laboratory. The timing of lights off was determined by participants’ habit, and the awakenings were spontaneous. If participants needed one parent had the possibility to remain and sleep in the same room on another bed for the whole night. Electroencephalograms (EEG) according to the 10–20 system (Jasper, 1958) at 18 recording sites (Fp1, Fp2, F3, F4, F7, F8, Fz, C3, C4, Cz, P3, P4, T3, T4, T5, T6, O1, O2) referred to the mathematically linked mastoids, left and right electrooculograms (EOG) with electrodes placed at the left and right outer canthi (contralateral mastoid reference), respectively, as well as bipolar submental electromyograms (EMG), electrocardiograms (ECG), and accelerometry-based left and right leg movement detections were carried out. Gold-coated Ag/AgCl electrodes fixed with EC2 Grass Electrode Cream (Grass Technologies, USA) served for EEG recordings, while EOG, EMG and ECG recordings were performed with disposable electrodes (T40/80, Telic S.A., Spain). Impedances for the EEG electrodes were kept below 5 kV. Signals were collected, prefiltered, amplified and digitized at a sampling rate of 249 Hz/channel by using the 30 channel Flat Style SLEEP La Mont Headbox with implemented second order filters at 0.5 Hz (high pass) and 70 Hz (low pass) as well as the HBX32-SLP 32 channel preamplifier (La Mont Medical Inc., USA). An additional 50 Hz digital notch filtering performed by the DataLab acquisition software (Medcare, Iceland) was carried out before data conversion (EDF, Kemp, Va¨ rri, Rosa, Nielsen, & Gade, 1992) and analysis.
2.1.3. Data analysis
Sleep recordings were visually scored according to standard criteria (Rechtschaffen & Kales, 1968) in 20 s epochs. Next the 4 s epochs containing artifacts were manually removed before further automatic analyses. Average power spectral densities were calculated by a mixed-radix Fast Fourier Transformation (FFT) algorithm applied to the 50% overlapping, Hanningtapered, artifact-free 4 s epochs of whole night stages 2–4 NREM sleep.
Both the raw values and the z-scores of 8–16 Hz spectra were used in the statistical analyses. z-Transformation was introduced in order to follow previous approaches in the field (De Gennaro et al., 2005) as well as to control for potentially simultaneous differences in general EEG amplitude and delta power, the latter being supported by our own results (Gombos et al., 2011) and indirectly by the reports of an increased SWS in WS (Gombos et al., 2011; Mason et al., 2008). The ztransformation results in series with a mean of 0 and standard deviation of 1, varying between 8 and 16 Hz, and reflecting the individual- and derivation-specific shapes of the spectra.
Spectral peaks were processed automatically as follows: the zero-crossing points of the first order derivatives of the spectra were considered as locations of spectral peaks if the second order derivativeswere negative at these frequencies (localmaxima in mathematical terms). Spectral peak processing was performed on the averaged z-scores of the left and right frontal (F3 and F4), aswell as on the left and right parietal (P3 and P4) derivations. As slower and faster sigmapeaks are usually dominant over the frontal and parietal derivations, respectively, we considered a spectral peak as frontally dominant if the averaged F3 and F4 z-score exceeded the averaged P3 and P4 z-score at the peak frequency, while the reverse was the criteria for parietally dominant peaks. Besides topography, the number and the frequencies of spectral peaks are reported here.
In order to test the differences in the distribution of NREM sleep 8–16 Hz EEG power along the antero-posterior cortical axis sagittally and parasagittally derived z-transformed power values were averaged [Fp = mean (Fp1, Fp2), F = mean (F3, F4, Fz), C = mean (C3, C4, Cz), P = mean (P3, P4), O = mean (O1, O2)] and then serially subtracted as follows: Fp F, F C, C P, P O. Positive outputs expressed power values with relative anterior, while negative ones with relative posterior dominance at the given frequency bin and region.
Statistical analyses included group comparisons by one-way analyses of variance (ANOVA).
2.2. Results
The comparisons of raw EEG power values by one-way ANOVA revealed WS-specific ranges of decreased power between the frequency limits of 9–13 Hz with 95% uncontrolled probabilities in almost all derivations. The modal frequencies of the lower and upper limits of power decrease were 10.50 and 12.50 Hz, respectively. No clear region specificity was observed (Fig. 1A). WS-dependent increases in power were observed at the vertex, at recording sites Cz (14.75–15.75 Hz) and C4 (15.25 Hz, see Fig. 1A for quantitative details).
Similar differences were reflected by the results of the comparisons of z-scores, revealing WS-specific decreases and increases in lower (9.25–13 Hz) and higher (13.75–16 Hz) sigma ranges, respectively. The modal frequency limits of the ranges with WS-specific power decrease and increase were 10.25–12.25 Hz and 14–16 Hz, respectively. This modal pattern of WS-specific alteration in power distribution was most consistently observed fronto-centro-parietally (derivations F3, F4, Fz, C3, C4, Cz, P3 and P4). Less consistent results were noted over the temporo-occipital regions (Fig. 1B).
As regarding the frontally detected spectral peaks 7 and 2 participants of the WS group had 1 and 2 detected peaks, respectively. These participants were 4 and 5 in the TD group (x2 = 2.10, p = .14). The groups differed in the fronto-parietal dominance of the spectral peaks detected in the frontal derivations (difference between the z-scores of the frontal and parietal derivations at the peak frequencies). Only two of the 11 peaks detected in the frontal channels of the WS groups showed frontal dominance (frontal > parietal). The remaining 9 were in fact the anterior appearances of the parietal peaks (frontal < parietal). The picture was different in the TD group, where 8 of the 14 frontally detected peaks showed a frontal dominance (x2 = 3.90, p = .048). Given the low number of frontally dominant spectral peaks it was impossible to statistically compare their frequencies.
The spectral peak analysis of the parietal derivations revealed a somewhat different picture. As in the case of frontally detected peaks the number of the peaks was not significantly different among the groups: one spectral peak was found in 6 and 7 participants of the TD and WS groups, respectively. Thus, 3 TD and 2 WS participants were characterized by 2 peaks (x2 = .28, p = .59). However, in contrast to the frontally detected peaks there was no group difference in the fronto-parietal dominance. Nine of 11 parietally detected peaks in the WS group and 10 of 12 peaks in the TD group were parietally dominant (x2 = .01, p = .92). That is, most of the parietally detected peaks were characterized by a parietal predominance in both groups. In order to compare the groups in terms of frequency of the parietally detected spectral peaks one peak frequency was assigned to each participant by selecting the peak with higher frequency for those participants characterized by 2 parietal peaks (3 TD and 2WS participants). The difference in the frequency of the fast parietal sigma peak of TD and WS participants (13.27 0.34 vs. 14.19 0.71, respectively) was significant (F = 12.03; df = 1, 16; p = .003).
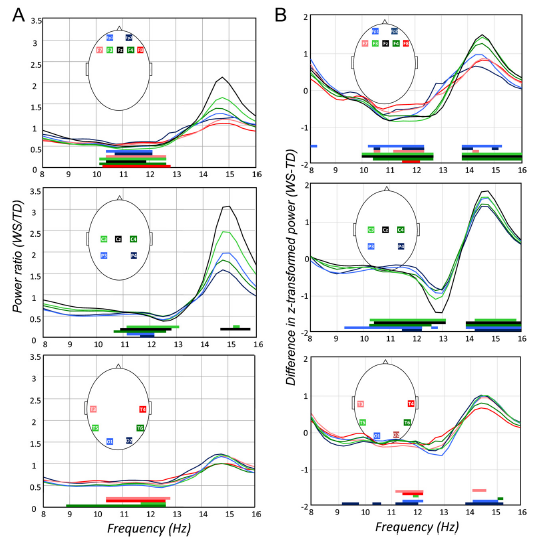
Fig. 1. Laboratory-recorded NREM sleep 8–16 Hz EEG power and z-score alterations in WS. Plots depict region (frontal, centro-parietal and temporooccipital)- and derivation-specific deflections of WS EEG power and z-score values from the TD ones. Significant group differences are shown by horizontal bars above the frequency axes with colour codes corresponding to the ones used for the EEG derivations. (A) NREM sleep 8–16 Hz EEG power ratios: WS (N = 9) over TD (N = 9). Note the decreased lower frequency (9–13 Hz) activity in all regions and the increased 14.75–15.75 Hz activity in the central region. (B) Differential z-scores of NREM sleep 8–16 Hz EEG power: WS (N = 9)minus TD (N = 9). Note the decreased lower frequency (9.25–13 Hz) activity and the increased higher frequency (13.75–16 Hz) activity in all regions with modal frequency limits of 10.25–12.25 Hz and 14–16 Hz. NREM, non-rapid-eye movement; WS, Williams syndrome; TD, typically developing.
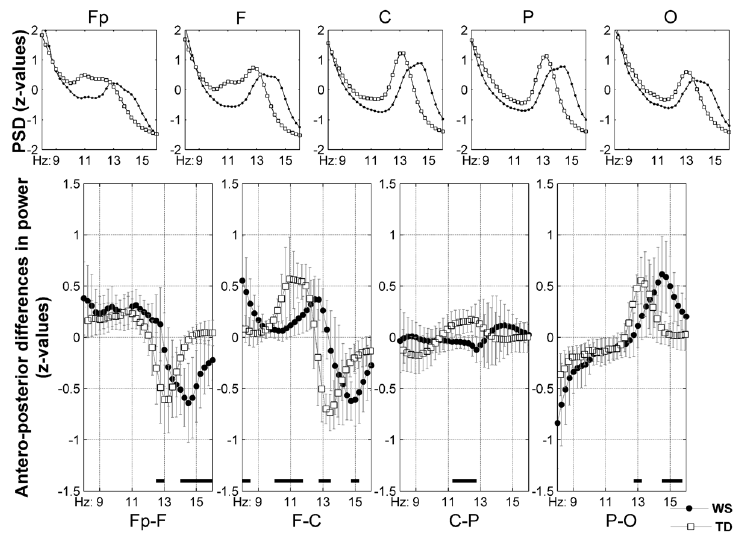
Fig. 2. (Para)sagittal means and antero-posterior differences in the z-scores of laboratory-recorded NREM sleep 8–16 Hz EEG power. Plots in the first row are group averages of individual means as follows: Fp =mean (Fp1, Fp2), F = mean (F3, F4, Fz), C = mean (C3, C4, Cz), P = mean (P3, P4), O = mean (O1, O2). The second row contains serial subtractions of the individual means: plotted are group means, vertical bars denote 95% confidence intervals (WS, closed circles; TD, open rectangles). Frequencies characterized by significant differences are shown by black horizontal bars over the frequency axes of the plots in the second row. Note the 1 Hz shifts in the frequency-dependence of antero-posterior distribution in the Fp–F, F–C and P–O comparisons. A lack of an anteroposterior power gradient at the centro-parietal (C-P) region in WS is also evidenced. PSD, power spectral density; NREM, non-rapid-eye movement; WS, Williams syndrome; TD, typically developing.
The antero-posterior distribution of z-transformed power is characterized by a predominance of lower frequency scores at the anterior and a predominance of the higher frequency scores at the posterior sites (Fig. 2). These tendencies covered the frontopolar-to-parietal regions in TD participants and the frontopolar-to-central regions in WS participants. Thus, WS participants failed to have a centro-parietal gradient in lower sigma activity in contrast to TD participants who had it. The parieto-occipital z-score distribution was characterized by a predominance of higher sigma values in the parietal as compared to the occipital derivations in both groups. The significant group differences in the antero-posterior power distributions are suggestive for a higher frequency at which the antero-posterior shifts occur (Fig. 2). This is supported by the visually observable differences in the peak frequencies of the group means of series Fp, F, C, P, and O (WS > TD; Fig. 2), as well as by the cross-correlation functions of group means in series Fp–F, F–C and P–O, which reached their maxima at 1 Hz delay, suggesting a higher frequency of WS participants’ sleep spindling.
2.3. Discussion
Evidence from our current investigation suggests that WS participants do not only have a disturbed sleep pattern (Arens et al., 1998; Gombos et al., 2011; Mason et al., 2008), but also a peculiar power distribution in their 8–16 Hz NREM sleep EEG. As massive experimental changes in sleep structure did not influence these individual patterns of z-transformed EEG power values (De Gennaro et al., 2005), we suggest that our main results are not the simple reflections of the increased SWS and decreased REM sleep and sleep efficiency of WS participants. The redistribution of the 8–16 Hz EEG power toward the higher frequencies was found to be a characteristic feature of our WS group in comparison with the TD one. This was evident by the region-independent decrease in the high alpha/low sigma (10.50–12.50 Hz) and the centrally increased high sigma (14.75– 15.75 Hz) raw EEG power (Fig. 1A). The z-scores of power values, which are the core measures expressing the EEG fingerprint of sleep, suggested the same redistribution characterized by decreased 10.25–12.25 Hz values and increased 14–16 Hz values in a region-independent manner, but with maximal effects at the fronto-centro-parietal derivations surrounding the vertex (Fig. 1B). The WS participants’ tendency for higher frequency activities was further supported by the significant WS > TD differences in parietal fast sigma peak frequencies. Moreover, the antero-posterior differences in power distributions occurred at a higher frequency in the WS as compared to the TD group (Fig. 2). Last, but not least, a significantly higher number of WS than TD participants lacked a frontally dominant spectral peak (the so-called low sigma activity) in their 8–16 Hz NREM sleep EEG. Thus, our results supported both of our hypotheses: a set of WS-specific features of the sleep EEG fingerprints were found and the distribution of NREM sleep 8–16 Hz EEG power along the antero-posterior cortical axis distinguishedWS from TD participants. Obviously there is need for precaution and further confirmations before generalizing our results. Reasons for this are some potential shortcomings of our study related to the use of a large number of statistical comparisons between the groups. An additional problem could be the lack of matching of our female participants with regard to the phase of their menstrual cycle, which affects the upper frequency range of sleep spindles (Driver, Dijk, Werth, Biedermann, & Borbély, 1996). Moreover, results of our hypothesis-driven study should be combined with major outcomes of other methods suitable for unravelling the genetic underpinnings of sleep EEG activities, including the analyses of quantitative trait loci in inbred strains of mice (Franken, Malafosse, & Tafti, 1998) as well as the study of subgroups of WS patients with partial deletions. The former approach proved to be fruitful in identifying strains with uncommon time courses of 11–19 Hz activities, but the absolute differences in frequencies were not yet reported (Franken et al., 1998).
3. Study II: sleep EEG fingerprints according to ambulatory sleep recordings of participants with WS
3.1. Introduction
The number of participants was relatively low in our first study. Consequently, we aimed to perform additional recordings on a larger sample of WS participants and healthy TD persons. These recordings are needed to be conduct in order to conclude that the reported differences reflect a sleep EEG fingerprint. Our further aim were to test the stability of the WSrelated sleep EEG pattern described in Study I by the inclusion of the 9WS participants and 3 from the TD participants of the sample in Study II. Finally, we aimed to control the possible effects of the laboratory environment by using ambulatory home polysomnography.
3.2. Methods
3.2.1. Participants
WS participants examined in Study I were followed up after 1 year (9 participants). Moreover, the study population was extended by including 11 further WS participants together with 11 TD controls. All WS participants (N = 20, 7 males and 13 females, age range 6–29 years, mean age 19.6 7.066 years) were contacted through the Hungarian Williams Syndrome Association. TD controls (N = 20, 6 males and 14 females, age range 6–29 years, mean age 19.6 7.007 years) were selected by personal contacts and matched by age and sex to theWSparticipants. The twin pair discordant forWSand sex examined in Study I was again considered as a pair case control.
3.2.2. Procedures and recordings
Home sleep was recorded according to the participants preferred sleeping habits by using a 32 channel SD-LTM Hardware together with the BRAIN QUICK SystemPLUS EVOLUTION software (Micromed, Italy). We recorded EEG according to the 10– 20 system (Jaspers, 1958) at 21 recording sites (Fp1, Fp2, Fpz, F3, F4, F7, F8, Fz, C3, C4, Cz, P3, P4, Pz, T3, T4, T5, T6, O1, O2, Oz) referred to the mathematically linked mastoids. Bipolar EOG, ECG and submental as well as tibialis EMG were also recorded. Signals were high-pass filtered at 0.33 Hz and low-pass filtered at 1500 Hz by a 40 dB/decade anti-aliasing hardware input filter. Data were collected with 22 bit resolution and with an analogue to digital conversion rate of 4096 Hz/channel (synchronous). A further 40 dB/decade anti-aliasing digital filter was applied by digital signal processing which low-pass filtered the data at 124 Hz. After this the digitized and filtered EEG was subsequently undersampled at 1024 Hz. A 50 Hz digital notch filtering performed by the recording software was also used. Data was exported and converted to EDF (Kemp et al., 1992) before further analyses.
3.2.3. Data analysis
Sleep recordings were analysed according to the methods and procedures presented in Study I, with the exception of using FFT instead of mixed-radix FFT on 4 second (4096 points) epochs. As there is no report on home polysomnographic features of participants withWSvisually scored sleep variables (Rechtschaffen & Kales, 1968) and sleep EEG power spectra in the classical frequency bands delta (0.5–4.5 Hz), theta (4.75–7.25 Hz), alpha (7.25–10.75 Hz), sigma (11–15 Hz), beta (15.25– 30 Hz), as well as gamma (30.25–45 Hz) are provided. Moreover, we analysed 21 EEG derivations instead of 18. As a consequence the analyses focusing on the antero-posterior gradients of sleep-EEG spectra included three more sagittal recording sites (Fpz, Pz and Oz) relative to Study I [Fp = mean (Fp1, Fp2, Fpz), F = mean (F3, F4, Fz), C = mean (C3, C4, Cz), P = mean (P3, P4, Pz), O = mean (O1, O2, Oz)]. These analyses were completed with the test of the stability of NREM sleep 8– 16 Hz EEG profiles, by calculating matrix correlations within and between-participants. Matrixes were the 8–16 Hz NREM sleep EEG spectral profiles expressed in z-scores (columns: EEG sites common in Study I and Study II, rows: frequency bins). The matrix correlations were Fisher z-transformed (zF), then averaged (Silver & Dunlap, 1987) for the within participant correlations (12 correlations according to the 1-year follow-up: zF-within) and between participant correlations (264 correlations, that is all possible combinations of different nights excluding the different nights of the same participants: zF-between). The mean within- and the mean between participant correlation were compared by the following formula: z = (zF-within – zF-between)/[1/(Nwithin – 3) + 1/(Nbetween – 3)]0.5, Nwithin and Nbetween being 12 and 264, respectively. The table of z-statistics was used to assess the significance of the difference between the mean within and between-participant correlations. Moreover, zF-within and zF-between were back-transformed to r values (Silver & Dunlap, 1987), and then squared, in order to estimate the difference between the variance explained by within- and between-participant similarity, respectively.
Statistical comparisons were performed by using independent-sample t-tests. Cases of inequality of variances were treated by using a d-test instead of the Student t.
3.3. Results
WSparticipants had higher sleep latency, more wake time after sleep onset, lower sleep efficiency, higher NREM and slow wave sleep percent and lower REM sleep percent. Spectral analysis revealed significant alterations in band power values of WS participants: frontopolar increases in delta power as well as more or less global decreases in alpha, sigma, beta and gamma power.
The results of the bin-to-bin analysis of the 8–16 Hz NREM sleep EEG were in line with the band power differences. WSspecific ranges of decreased power between the frequency limits of 8–12.5 Hz were observed with 95% uncontrolled probabilities in all EEG derivations. In the EEG derivations F7, F8, and T3 the significant WS-specific decrease was extended over the higher sigma ranges up to 15 or even 16 Hz. The modal frequencies of the lower and upper limits of power decrease were 8 and 11.75 Hz, respectively (Fig. 3A). Another frequency domain characterized by continuous WS-specific decrease in power was the one between 12.5 and 16 Hz. In this case the modal frequencies of lower and upper limits of power decrease were 13.75 and 16 Hz, respectively (Fig. 3A). The power decrease with modal frequency limits of 13.75–16 Hz is present in frontal, temporal and occipital regions, but not in the central and parietal ones.
The reliability of the z-scores of the 8–16 Hz NREM sleep EEG spectra was tested by using matrix correlations. Mean within- and between participant correlations (Fisher-z transformed, averaged and back-transformed) were 0.94 and 0.61, respectively. Thus, 89% and 37% of the variance was explained by within- and between participant similarity, respectively. In other words the individual-specific 8–16 Hz NREM sleep EEG profile explains somewhat more than 50% (89–37) of the variance. The difference between mean within- and between participant zF correlations was significant (z = 3.13; p = .0009, one-tailed).
The z-scores of the 8–16 Hz NREM sleep EEG spectra were significantly different among the groups, revealing WS-specific decreases and increases in lower (8–11.75 Hz) and upper (13–16 Hz) sigma ranges, respectively. The modal frequency limits of the ranges with WS-specific power decrease and increase were 8.50–11.25 Hz and 13.5–14 Hz, respectively (Fig. 3B). The number of the spectral peaks detected in the frontal derivations was equally distributed among the groups: 16 WS and 13 TD participants had one frontal peak, while 3 WS and 7 TD participants had 2 frontal peaks. 1 WS participant had 3 frontal peaks, and was included among the participants with 2 peaks (x2 = 1.13, p = .28). However, only 10 out of the 25 frontal peaks in the WS group showed frontal dominance, while 15 peaks were characterized by a parietal predominance. This contrasted the findings regarding the fronto-parietal dominance of the spectral peaks in the TD group: among the 26 frontally detected peaks 20 were frontally dominant also, while only 6 showed a parietal dominance (x2 = 7.17, p = .007). The spectral peak frequency (the frequency at which the peaks were detected) was compared among the groups by assigning one peak to each participant. This was the slowest peak in those participants having more than 1 frontal peak (low sigma activity). WS participants had significantly higher spectral peak frequency in their frontal derivations (12.97 0.99 vs. 12.06 1.17; F = 6.85; df = 1, 37; p = .012).
As in the case of the frontally located peaks, the number of the spectral peaks detected in the parietal derivations was equal in the groups: 17 WS and 17 TD participants had 1 parietally detected peak, while 3WS and 3 TD had 2. There was no group difference in the fronto-parietal dominance of the parietally detected peaks as most of the peaks were parietally dominant in both groups: 15 of 23 and 16 of 23 peaks in the WS and TD groups, respectively (x2 = .10, p = .75). Although, the peak frequency of the fastest parietal peaks (high sigma activity) differed among the groups (13.81 0.79 in WS vs. 13.51 0.65 in TD), the difference was not significant (F = 1.71; df = 1, 38; p = .19).
The antero-posterior distribution of the z-scores of 8–16 Hz NREM sleep EEG spectra is characterized by a predominance of lower frequency values at the anterior and a predominance of higher frequency values at the posterior sites (Fig. 4). These tendencies covered the frontopolar-to-parietal regions in both WS and TD participants. The parieto-occipital z-score distribution was characterized by a predominance of higher sigma scores in the parietal as compared to the occipital derivations in both groups. The significant group differences in the antero-posterior z-score distributions are suggestive for higher anterior-to-posterior differences at lower and higher frequencies in Fp–F and P–O series in WS participants in comparison with TD participants (Fig. 4). Moreover, the higher frequency at which the antero-posterior shift occurs is evident in several series (Fig. 4) and supported by the maxima in the cross-correlation functions occurring at 0.5 Hz delay in series Fp–F and F–C.
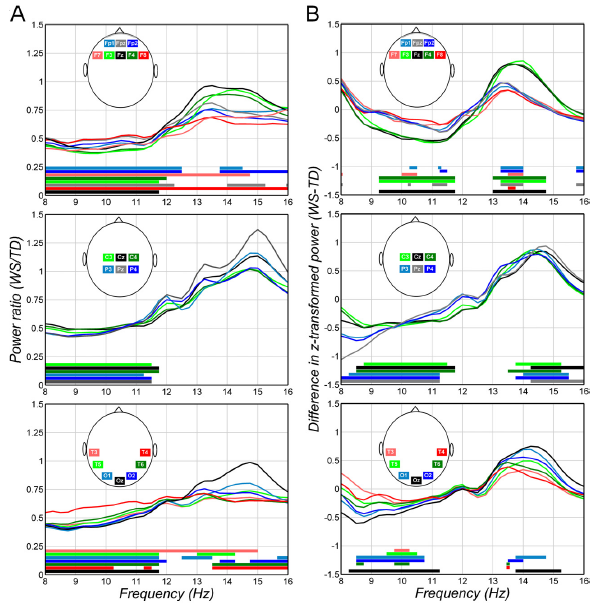
Fig. 3. Home-recorded NREM sleep 8–16 Hz EEG power and z-score alterations in WS. Plots depict region (frontal, centro-parietal and temporo-occipital)- and derivation-specific deflections of WS EEG power and z-score values from the TD ones. Significant group differences are shown by horizontal bars above the frequency axes with colour codes corresponding to the ones used for the EEG derivations. (A) NREM sleep 8–16 Hz EEG power ratios: WS (N = 20) over TD (N = 20). Note the decreased lower frequency (8–12.5 Hz) activity in all regions and the decreased 12.5–16 Hz activity in several derivations of the frontal, temporal and occipital, but not central and parietal regions. (B) Differential z-scores of NREM sleep 8–16 Hz EEG power: WS (N = 20) minus TD (N = 20). Note the decreased lower frequency (8–11.75 Hz) activity and the increased higher frequency (13–16 Hz) activity in all regions with modal frequency limits of 8.50–11.25 Hz and 13.5–14 Hz. NREM, non-rapid-eye movement; WS, Williams syndrome; TD, typically developing.
3.4. Discussion
WS participants were characterized by alterations in sleep architecture. These alterations were in line with our previous findings based on the laboratory records of a smaller sample (Gombos et al., 2011), as well as with the two other reports in the literature (Arens et al., 1998; Mason et al., 2008). That is WS participants had lower sleep efficiency more wakefulness and slow wave sleep, as well as less REM sleep than their TD controls.
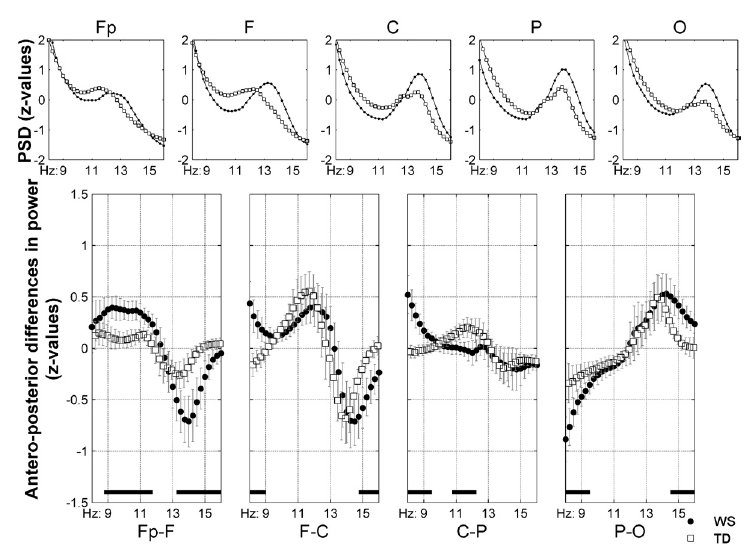
Fig. 4. (Para)sagittal means and antero-posterior differences in the z-scores of home-recorded NREM sleep 8–16 Hz EEG power. Plots in the first row are group averages of individual means as follows: Fp =mean (Fp1, Fp2, Fpz), F = mean (F3, F4, Fz), C = mean (C3, C4, Cz), P = mean (P3, P4, Pz), O = mean (O1, O2, Oz). The second row contains serial subtractions of the individual means: plotted are group means, vertical bars denote 95% confidence intervals (WS, closed circles; TD, open rectangles). Frequencies characterized by significant differences are shown by black horizontal bars over the frequency axes of the plots in the second row. Note the 0.5 Hz shifts in the frequency-dependence of antero-posterior distribution in the Fp–F and F–C comparisons. PSD, power spectral density; NREM, non-rapid-eye movement; WS, Williams syndrome; TD, typically developing.
The band power values of the NREM sleep EEG spectra of WS participants were significantly different from the TD group in terms of delta (increase), as well as alpha, sigma, beta and gamma (decreases) activities. The delta, the alpha and the sigma alterations are cohering with our previous findings (Gombos et al., 2011). The decreased NREM sleep beta EEG power values were not reported in Study I. The possibility exists that this difference between the findings is related to the relatively low sample size of Study I, but we cannot exclude the possibility that some WS-specific differences are age-specific, and hence different in the sample including children (Study II). Moreover, the significant group differences are not confined to the beta range, but cover the gamma band also. In summary, both laboratory and home sleep recordings reveal a list of similar alterations in macrostructural (decreased sleep efficiency, decreased REM sleep percent, increased wakefulness and slow wave sleep) and NREM EEG power spectral measures (increased frontal delta, decreased alpha and sigma) of sleep in WS. It remains to be determined whether higher frequency EEG activities are consistently reduced in participants with WS.
The 8–16 Hz NREM sleep EEG spectra of WS participants was characterized by decreased values between the modal frequency limits of 8–11.75 Hz and 13.75–16 Hz. This is in line with the band power results reported before (see also Gombos et al., 2011), but is partially dissimilar with results of our findings of Study I in the present report. The 10.50–12.50 Hz range was the one characterized by decreased activity in Study I, whereas the 8–11.75 Hz range in Study II. Moreover, there were some frequency bins (14.75–15.75 Hz) and locations (central electrodes) with increased WS-specific values in Study I, but these were not replicated in Study II. A decreased activity covering this frequency range (13.75–16 Hz) was reported in frontal, temporal and occipital, but not central and parietal locations instead. These dissimilarities could be results of the larger age range of our participants in Study II (6–29 years) when compared to Study I (14–29 years). Therefore, the common finding of our two studies in this regard is that of a decreased NREM sleep EEG activity in the 10–11.75 Hz range, which is the overlap of the two frequency ranges and could be considered as a common denominator of the two studies in this regard. Moreover, this is in line with the decreased NREM sleep EEG alpha band power of WS participants reported previously (Gombos et al., 2011).
As regarding the z-scores of the 8–16 Hz NREM sleep EEG spectra, these were much more confirmative. The main findings of Study I were the WS-specific decreases and increases between 10.25–12.25 Hz and 14–16 Hz, respectively. Results of Study II revealed a similar pattern of WS-specific z-score decreases followed by increases between the limits of 8.50– 11.25 Hz and 13.5–14 Hz. Thus, a decrease around 10–11.25 Hz and an increase around 14 Hz is supported by both studies. Moreover, the 1-year follow-up of the subsample containing 9WS and 3 TD participants (N = 12) revealed a striking stability of the spectral profiles of the 8–16 Hz NREM sleep EEG. This is coherent with previous reports in the literature (De Gennaro et al., 2005) and provides further support for our approach aiming to reveal stable differences between the groups.
The NREM sleep EEG spectral peaks in the 8–16 Hz range were different in their dominancy. A considerable higher number of WSparticipants showed just an extension of their parietally dominant peaks over the frontal area, without having a frontally dominant (so-called low sigma or slow spindle) peak. This statement and other results regarding the number and dominancy of the peaks cohere with the findings of Study I. There was also an evidence for a higher peak frequency of the WS participants in their frontal EEG derivations, but not in the parietal ones.
The antero-posterior differences in the NREM sleep 8–16 Hz EEG power distributions as revealed by the subtractions of zscores occurred at a higher frequency in the WS than in the TD group. This was evident from the visual inspection of Fig. 4, as well as from the cross-correlation functions between the antero-posteriorly subtracted mean series of theWSand TD groups, revealing roughly a 0.5 Hz phase shift between the groups. This result as well as the visually discernible differences in the peak frequencies of group means of series Fp and F (Fig. 4) are replications of our corresponding finding in Study I, as well as a direct evidence for the fact that the 8–16 Hz NREM sleep EEG of bothWSand TD groups can be divided into components with anterior and posterior dominance, but the border between the two is of higher frequency in the WS participants. We also revealed a significantly larger antero-posterior difference in z-scores in the WS participants in some regions. Reasons for this are completely unknown at the moment and we could only speculate on the origins of these differences as well as on their apparent lack in the results of Study I.
4. Overall discussion
The 8–16 Hz frequency range and the antero-posterior cortical axis are two dimensions characterizing the individual profile of the NREM sleep EEG power spectra. Like a ‘‘fingerprint’’ this profile is substantially invariant across several consecutive nights despite of large experimentally induced changes in sleep architecture, being strongly influenced by genetic factors (De Gennaro et al., 2005, 2008). On the basis of the evidence for the trait-like characteristics in the sleep EEG, a need for further studies identifying distinct phenotypes and specific genes underlying functional aspects of human sleep was expressed (Buckelmu¨ ller et al., 2006). Our results are potentially interesting in this regard as the genes which are deleted in WS participants during meiosis were unequivocally identified, thus the study of WS affords a privileged setting to understand genetic influences on complex brain functions such as neuronal oscillations and sleep in a bottom-up way (Dai et al., 2009; Meyer-Lindenberg et al., 2006).
As there is substantial evidence supporting the theory that thalamocortical resonance is a neurophysiologic mechanism of NREM sleep EEG spindles (Huguenard & McCormick, 2007; Steriade, 2000), our results suggest an accelerated frequency of the bursting thalamocortical oscillatory dynamics in WS participants. We can only speculate on the possible reasons of this acceleration. One possible line of reasoning could be the one related to the genetically determined differences in gross brain anatomy as a cause of this peculiar feature. The brains of children with WS have a decreased volume of the right parietooccipital regions and basal ganglia (Campbell et al., 2009). Basal ganglia dysfunctions ofWSparticipants are supported by the presence of mild extrapyramidal signs (Gagliardi, Martelli, Burt, & Borgatti, 2007). The source of a potent regulatory control of the thalamocortical circuitry lies in the basal ganglia. Basal ganglia dysfunctions in patients with Huntington disease might cause thalamocortical hyperactivity and an associated increase in sleep spindling, but the frequency of spindles were not characterized in those studies (Wiegand et al., 1991). Although potential increases in spindle density was not tested in our current study, specific alterations in basal ganglia functioning might cause an accelerated frequency of thalamocortical resonance during the NREM phases of sleep in WS participants. Further support for this line of reasoning comes from studies unravelling distinct genes that affect the 8–16 Hz range of the NREM sleep EEG (Bodenmann et al., 2009; Rétey et al., 2005). Although, the reports consider genes outside of the 7q11.23 region, some of those genes may also affect basal ganglia– thalamocortical circuitry by influencing dopaminergic transmission.
At the cellular level sleep spindling was shown to emerge from the synchronized hyperpolarization-rebound sequences of the thalamocortical neurons, the inhibition of which is caused by the NREM-dependent activation of GABAergic neurons in the reticular thalamic nucleus (Huguenard & McCormick, 2007; Steriade, 2000). Genetically determined alterations in specific receptors and/or ion channels within these complex thalamo-cortico-thalamic loops might cause an increase in the preferred resonance frequency of the whole system also. The particularly large variance in the sleep spindle frequency of WS participants calls for a need of corroborating genetic analyses with individualized quantitative sleep EEG measures, as subgroups of WS patients were shown to have smaller than usual deletions in chromosome 7q11.23 (Meyer-Lindenberg et al., 2006). Partial deletions may help to narrow down the list of candidate genes for the sleep EEG phenotype. Indirect evidence for the strong genetic influences on sleep polysomnography and microarchitecture comes from studies reporting striking similarities in sleep phenotypes of twins discordant for chronic fatigue syndrome (Armitage et al., 2009).
A third possible physiological basis of the acceleration of NREM sleep thalamocortical oscillatory dynamics could be a hormonal dysfunction during the sleep period of participants with WS. There are preliminary reports suggesting the possibility of a dysfunctional melatonin release in WS participants (Sniecinska et al., 2010). Moreover, those sleep periods associated with low melatonin levels (e.g. daytime sleep in forced desynchrony protocols) were shown to be characterized by an accelerated sleep spindle/sigma activity (Dijk, Shanahan, Duffy, Ronda, & Czeisler, 1997). Hence, a pineal or circadian dysfunction could be a common basis for both sleep disorders and EEG changes of participants with WS.
Another issue of potential interest in analyzing the sleep EEG fingerprint of patients withWSis the possibility to study the relationship between the behavioral/cognitive and the sleep-EEG phenotype. This possibility is strengthened by previous studies reporting WS-specific alterations in visuospatial constructive abilities and motor learning (Dai et al., 2009; Meyer- Lindenberg et al., 2006), as both were shown to be related to individual features of sleep EEG spindling activity in healthy volunteers (Bódizs, Lázár, & Rigó, 2008; Morin et al., 2008). However, we cannot exclude the possibility that the differences between patients and TD controls may reflect higher sleep debt in WS, possibly related to their ‘‘hypersociability’’ (Meyer- Lindenberg et al., 2006). In this case specific sleep-EEG changes could be indirect results of the WS-specific behavioral phenotype.
5. Conclusions
In conclusion, our results provide evidence for the existence of a distinct phenotype of NREM sleep EEG 8–16 Hz activity found to be characteristic for WS. This phenotype is characterized by an increased frequency of sleep spindle activity and/or a redistribution of alpha/sigma activities toward the higher frequency end of the spectrum together with the characteristic reorganization of the frequency topography relationship. The 25–28 genes which are deleted in WS are among the candidates of regulators of sleep spindle frequency in particular and of shapers of the sleep EEG fingerprint in general. A corroboration of our results with the compelling evidences suggesting that bursting thalamocortical oscillations are the neurophysiological bases of sleep spindling (Huguenard & McCormick, 2007; Steriade, 2000), these issues point to an accelerated NREM sleep-dependent thalamocortical oscillatory dynamics in WS.
Acknowledgements
This work was supported by the Hungarian National Science Found (OTKA-NF60806 to I.K.) and by TÁMOP-4.2.2.B-10/1– 2010-0009. Authors thank Péter Ujma for his assistance in data analysis.
References
Ambrosius, U., Lietzenmaier, S., Wehrle, R., Wichniak, A., Kalus, S., Winkelmann, J., et al. (2008). Heritability of sleep electroencephalogram. Biological Psychiatry, 64, 344–348.
Arens, R., Wright, B., Elliott, J., Zhao, H., Wang, P. P., Brown, L. W., et al. (1998). Periodic limb movement in sleep in children with Williams syndrome. The Journal of Pediatrics, 133, 670–674.
Armitage, R., Landis, C., Hoffmann, R., Lentz, M., Watson, N., Goldberg, J., et al. (2009). Power spectral analysis of sleep EEG in twins discordant for chronic fatigue syndrome. Journal of Psychosomatic Research, 66, 51–57.
Bellugi, U., Lichtenberger, L., Jones, W., Lai, Z., & St George, M. (2000). The neurocognitive profile of Williams Syndrome: A complex pattern of strengths and weaknesses. Journal of Cognitive Neuroscience, 12, S7–S29.
Bodenmann, S., Rusterholz, T., Du¨ rr, R., Stoll, C., Bachmann, V., Geissler, E., et al. (2009). The functional Val158Met polymorphism of COMT predicts interindividual differences in brain alpha oscillations in young men. The Journal of Neuroscience, 29, 10855–10862.
Bódizs, R., Lázár, A. S., & Rigó, P. (2008). Correlation of visuospatial memory ability with right parietal EEG spindling during sleep. Acta Physiologica Hungarica, 95, 297–306.
Bódizs, R., Körmendi, J., Rigó, P., & Lázár, A. S. (2009). The individual adjustment method of sleep spindle analysis: Methodological improvements and roots in the fingerprint paradigm. Journal of Neuroscience Methods, 178, 205–213.
Bruni, O., Ferri, R., Novelli, L., Terribili, M., Troianiello, M., Finotti, E., et al. (2009). Sleep spindle activity is correlated with reading abilities in developmental dyslexia. Sleep, 32, 1333–1340.
Buckelmu¨ ller, J., Landolt, H. P., Stassen, H. H., & Achermann, P. (2006). Trait-like individual differences in the human sleep electroencephalogram. Neuroscience, 138, 351–356.
Campbell, L. E., Daly, E., Toal, F., Stevens, A., Azuma, R., Karmiloff-Smith, A., et al. (2009). Brain structural differences associated with the behavioural phenotype in children with Williams syndrome. Brain Research, 1258, 96–107.
Dai, L., Bellugi, U., Chen, X. N., Pulst-Korenberg, A. M., Ja¨rvinen-Pasley, A., Tirosh-Wagner, T., et al. (2009). Is it Williams syndrome? GTF2IRD1 implicated in visualspatial construction and GTF2I in sociability revealed by high resolution arrays. American Journal of Medical Genetics: Part A, 149A, 302–314.
De Gennaro, L., Ferrara, M., Vecchio, F., Curcio, G., & Bertini, M. (2005). An electroencephalographic fingerprint of human sleep. Neuroimage, 26, 114–122.
De Gennaro, L., Marzano, C., Fratello, F., Moroni, F., Pellicciari, M. C., Ferlazzo, F., et al. (2008). The electroencephalographic fingerprint of sleep is genetically determined: A twin study. Annals of Neurology, 64, 455–460.
Dijk, D. J., Shanahan, T. L., Duffy, J. F., Ronda, J. M., & Czeisler, C. A. (1997). Variation of electroencephalographic activity during non-rapid eye movement and rapid eye movement sleep with phase of circadian melatonin rhythm in humans. The Journal of Physiology, 505(Pt 3), 851–858.
Driver, H. S., Dijk, D. J., Werth, E., Biedermann, K., & Borbély, A. A. (1996). Sleep and the sleep electroencephalogram across the menstrual cycle in young healthy women. The Journal of Clinical Endocrinology and Metabolism, 81, 728–735.
Feinberg, I., Fein, G., & Floyd, T. C. (1980). Period and amplitude analysis of NREM EEG in sleep: Repeatability of results in young adults. Electroencephalography and Clinical Neurophysiology, 48, 212–221.
Finelli, L. A., Achermann, P., & Borbély, A. A. (2001). Individual fingerprints in human sleep EEG topography. Neuropsychopharmacology, 25, S57–S62.
Franken, P., Malafosse, A., & Tafti, M. (1998). Genetic variation in EEG activity during sleep in inbred mice. The American Journal of Physiology, 275, R1127–R1137. Gaillard, J.-M., & Blois, R. (1981). Spindle density in sleep of normal participants. Sleep, 4, 385–391.
Gagliardi, C., Martelli, S., Burt, M. D., & Borgatti, R. (2007). Evolution of neurologic features in Williams syndrome. Pediatric Neurology, 36, 301–306.
Godbout, R., Bergeron, C., Limoges, E., Stip, E., & Mottron, L. (2000). A laboratory study of sleep in Asperger‘s syndrome. Neuroreport, 11, 127–130.
Goldman, S. E., Malow, B. A., Newman, K. D., Roof, E., & Dykens, E. M. (2009). Sleep patterns and daytime sleepiness in adolescents and young adults with Williams syndrome. Journal of Intellectual Disability Research, 53, 182–188.
Gombos, F., Bódizs, R., & Kovács, I. (2011). Atypical sleep architecture and altered EEG spectra in Williams syndrome. Journal of Intellectual Disability Research, 55(3), 255–262.
Huguenard, J. R., & McCormick, D. A. (2007). Thalamic synchrony and dynamic regulation of global forebrain oscillations. Trends in Neurosciences, 30, 350–356.
Jasper, H. H. (1958). Report of the committee on methods of clinical examination in electroencephalography. Electroencephalography and Clinical Neurophysiology, 10, 370–375.
Ja¨rvinen-Pasley, A., Bellugi, U., Reilly, J., Mills, D. L., Galaburda, A., Reiss, A. L., et al. (2008). Defining the social phenotype in Williams syndrome: A model for linking gene, the brain, and behavior. Development and Psychopathology, 20, 1–35.
Karmiloff-Smith, A., Grant, J., Berthoud, I., Davies, M., Howlin, P., & Udwin, O. (1997). Language and Williams syndrome: How intact is ‘intact’? Child Development, 68, 246–262.
Kemp, B., Va¨ rri, A., Rosa, A. C., Nielsen, K. D., & Gade, J. (1992). A simple format for exchange of digitized polygraphic recordings. Electroencephalography and Clinical Neurophysiology, 82, 391–393.
Leyfer, O. T., Woodruff-Borden, J., Klein-Tasman, B. P., Fricke, J. S., & Mervis, C. B. (2006). Prevalence of psychiatric disorders in 4–16-year-olds with Williams syndrome. American Journal of Medical Genetics. Part B: Neuropsychiatric Genetics, 141B(6), 615–622.
Mason, T., Arens, R., Sharman, J., Pack, A., & Kaplan, P. (2008). Polysomnography Findings in Children with Williams Syndrome. Frontiers in Human Neuroscience. Conference Abstract 12th International Professional Conference on Williams Syndrome, doi:10.3389/conf.neuro.09.2009.07.052.
Meyer-Lindenberg, A., Mervis, C. B., & Berman, K. F. (2006). Neural mechanisms in Williams syndrome: A unique window to genetic influences on cognition and behaviour. Nature Reviews. Neuroscience, 7, 380–893.
Morin, A., Doyon, J., Dostie, V., Barakat, M., Hadj Tahar, A., Korman, M., et al. (2008). Motor sequence learning increases sleep spindles and fast frequencies in posttraining sleep. Sleep, 31, 1149–1156.
Morris, C. A., & Mervis, C. B. (2000). Williams syndrome and related disorders. Annual Review of Genomics and Human Genetics, 1, 461–484.
Rechtschaffen, A., & Kales, A. (1968). Manual of standardized terminology, techniques and scoring system for sleep stages of human participants. Los Angeles: UCLA Brain Information Service/Brain Research Institute.
Rétey, J. V., Adam, M., Honegger, E., Khatami, R., Luhmann, U. F., Jung, H. H., et al. (2005). A functional genetic variation of adenosine deaminase affects the duration and intensity of deep sleep in humans. Proceedings of the National Academy of Sciences of the United States of America, 102, 15676–15681.
Selvitelli, M. F., Krishnamurthy, K. B., Herzog, A. G., Schomer, D. L., & Chang, B. S. (2009). Sleep spindle alterations in patients with malformations of cortical development. Brain & Development, 31, 163–168.
Silver, N. C., & Dunlap, W. P. (1987). Averaging correlation coefficients: Should Fisher’s z transformation be used? The Journal of Applied Psychology, 72(1), 146–148.
Silverstein, L. D., & Levy, C. M. (1976). The stability of the sigma sleep spindle. Electroencephalography and Clinical Neurophysiology, 40, 666–670.
Sniecinska, A., Butler, S., Iles, R., Bayford, R., Burczynska, B., & Annaz, D. (2010). Melatonin and sleep in typically developing children and children with Williams syndrome. Journal of Sleep Research, 19(Suppl. 2), 260.
Steriade, M. (2000). Corticothalamic resonance, states of vigilance and mentation. Neuroscience, 101, 243–276.
Tan, X., Campbell, I. G., Palagini, L., & Feinberg, I. (2000). High internight reliability of computer-measured NREM delta, sigma, and beta: Biological implications. Biological Psychiatry, 48, 1010–1019.
Tarokh, L., Carskadon, M. A., & Achermann, P. (2011). Trait-like characteristics of the sleep-EEG across adolescent development. The Journal of Neuroscience, 31, 6371–6378.
Wiegand, M., Mo¨ ller, A. A., Lauer, C. J., Stolz, S., Schreiber, W., Dose, M., et al. (1991). Nocturnal sleep in Huntington‘s disease. Journal of Neurology, 238, 203–208.
|